Exponential random graph models for little networks |
| |
Affiliation: | 1. Department of Preventive Medicine, University of Southern California, USA;2. US Army Research Institute for the Behavioral and Social Sciences, USA |
| |
Abstract: | 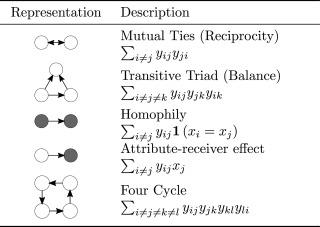 Statistical models for social networks have enabled researchers to study complex social phenomena that give rise to observed patterns of relationships among social actors and to gain a rich understanding of the interdependent nature of social ties and actors. Much of this research has focused on social networks within medium to large social groups. To date, these advances in statistical models for social networks, and in particular, of Exponential-Family Random Graph Models (ERGMS), have rarely been applied to the study of small networks, despite small network data in teams, families, and personal networks being common in many fields. In this paper, we revisit the estimation of ERGMs for small networks and propose using exhaustive enumeration when possible. We developed an R package that implements the estimation of pooled ERGMs for small networks using Maximum Likelihood Estimation (MLE), called “ergmito”. Based on the results of an extensive simulation study to assess the properties of the MLE estimator, we conclude that there are several benefits of direct MLE estimation compared to approximate methods and that this creates opportunities for valuable methodological innovations that can be applied to modeling social networks with ERGMs. |
| |
Keywords: | Exponential random graph models Small networks Exact statistics Simulation study Teams |
本文献已被 ScienceDirect 等数据库收录! |
|